A clinical prediction tool for mortality in ARDS
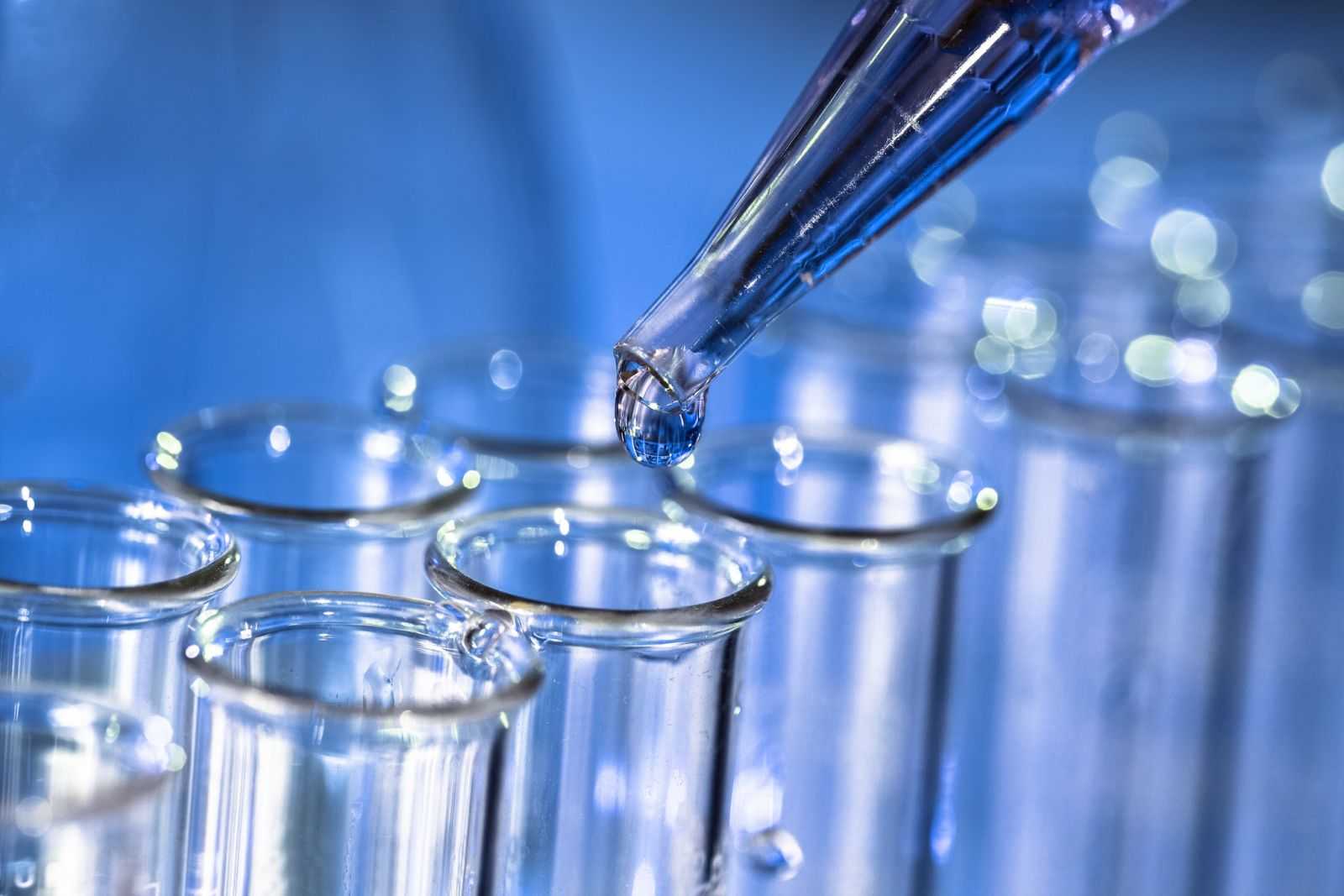
ICM ARTICLE REVIEW
Background
Acute respiratory distress syndrome (ARDS) is a heterogeneous entity with substantial incidence and mortality. Decades of investigation have failed to develop pharmacological therapies to improve its outcome. This might be a consequence of failure to identify the patients most suitable for treatment (i.e. with higher mortality), resulting in underpowered trials. There has been growing interest in developing predictive tools to allow more targeted therapies. Ware et al. [1] have previously developed a combined prognostic tool (including age, APACHE III score, and the levels of surfactant protein D and interleukin-8) that has not been externally validated.
Objective and methods
Zhao et al [2] aimed to validate this model in three ARDS cohorts: two randomised controlled trials (RCT): FACTT [3] and STRIVE [4]; and the subgroup of patients that met Berlin ARDS criteria in the VALID [5] cohort. Their secondary goal was to prove that the addition of biomarkers improved the predictive value of the model. Finally, they performed a prognostic enrichment analysis, by redefining two subgroups on the FACTT trial (based on predicted mortality lower or higher than 20%) and comparing differential treatment effect on ventilator free days (VFDs).
They included 1538 patients in the analysis, recalculated the APACHE III score based on a validated translation equation from APACHE II, and measured plasma biomarkers. Model discrimination was assessed by the area under the receiver operator characteristic curve (ROC AUC). Calibration and recalibration were assessed graphically, with predicted mortality in the x-axis and observed mortality in the y-axis.
They included 1538 patients in the analysis, recalculated the APACHE III score based on a validated translation equation from APACHE II, and measured plasma biomarkers. Model discrimination was assessed by the area under the receiver operator characteristic curve (ROC AUC). Calibration and recalibration were assessed graphically, with predicted mortality in the x-axis and observed mortality in the y-axis.
Results
There were significant differences between cohorts in the mortality rates, cause of ARDS, degree of organ dysfunction and distribution of biomarkers. This probably resulted in a ROC AUC lower than in the original cohort, but still performing well (0.74 in FACTT, 0.73 in VALID and 0.78 in STRIVE). The addition of biomarkers to the model improved the ROC AUC significantly. Calibration and recalibration showed how the model deviated at the highest levels of expected mortality (i.e. > 40%). FACTT subgroup analysis implied that fluid-restrictive treatment did only have a positive effect in the high-risk cohort of patients, being neutral in the lower-risk.
Strengths and weaknesses
- This is the first paper to successfully validate a prediction model in ARDS.
- Although the concordance of the predictive model was worse than previously reported, it is noteworthy that it allowed to define risk profile groups.
- On a post-hoc subgroup analysis, a correlation between high risk of mortality and effective intervention was suggested.
- The cohorts chosen for the analysis were heterogeneous and might not represent current ARDS patients. APACHE III score was calculated as a surrogate; the distribution of biomarkers was different than in the original cohort. All of these must have reduced the strength of the predictive model.
- However, heterogeneity may also enhance external validity.
Conclusion
A predictive model for mortality in ARDS has been validated in a wide range of patients. This could represent a valuable tool in designing future RCTs.
Article review was prepared and submitted by Joaquim Cevallos and NEXT Committee member Brijesh Patel on behalf of the EJRC.
REFERENCES
1. Ware LB et al (2010). Prognostic and pathogenetic value of combining clinical and biochemical indices in patients with acute lung injury. Chest 137: 288-296.
2. Zhao Z et al (2017). External validation of a biomarker and clinical prediction model for hospital mortality in acute respiratory distress syndrome. Intensive Care Med 43: 1123-1131.
3. Wiedeman HP et al (2006). Comparison of two fluid-management strategies in acute lung injury. N Engl J Med 351: 327-336.
4. Zeiher BG et al (2004). Neutrophil elastase inhibition in acute lung injury: results of the STRIVE study. Crit Care Med 32: 1695-1702.
5. O’Neal HR et al (2011). Prehospital statin and aspirin use and the prevalence of severe sepsis and acute lung injury/acute respiratory distress syndrome. Crit Care Med 39: 1343-1350.